
In other words, it's a way of asking yourself whether it is valid to use the mean to describe the whole population, or whether the information brought by the explanatory variables is of value or not. The results enable us to determine whether or not the explanatory variables bring significant information (null hypothesis H0) to the model. It is important to examine the results of the analysis of variance table (see below). The remaining 8 percent are hidden in other variables, which the model classifies as "random effects." In this particular example, 92% of the variability is explained. The coefficient of determination (0.92) gives us a fair idea of the extent to which the variability of the modeled variable (the yield) can be explained by the explanatory variables (the method, the type of field, and their interaction). The first results displayed by XLSTAT are the goodness of fit coefficients including the R’² (coefficient of determination), and the adjusted R’². Interpreting the results of a two-way unbalanced ANOVA with interactions A dialog box is displayed so that you can confirm which factors have to be taken into account in the model. The computations begin once you have clicked on the OK button. In the Outputs tab, the Type SS options were activated because we want the model to take the interactions into account, and because we want to analyze the F values given in the Type I SS, and Type III SS tables (SS stands for sum of squares). The only difference it makes is in the actual writing of the model. We left the constraint option at a1=0, meaning that we want the model to be built on the assumption that the method "1" has the standard effect on the yield.Īlthough you have to apply a constraint to the model in ANOVA for theoretical reasons, it will not affect the results (goodness of fit, predictions). The interactions option is activated on the options tab, and the maximum level of intraction is set to 2. The second way of selecting the data is in the form of a table, with, in columns, the modalities of an explanatory variable, and in lines, the modalities of the second explanatory variable. The first is in the form of columns, one column for the dependent variable, and the others for the explanatory variables. In XLSTAT, it is possible to select the data in two different ways for the ANOVA. Our aim is to determine the effect of the method, the type of field and the interaction between the two on the variability of the yield.Īs we selected the column title for the variables, we left the option Variable labels activated. The Dependent variable (or variable to model) is here the "Yield". Once you've clicked on the button, the ANOVA dialog box appears. Setting up a two-way unbalanced ANOVA with interactionsĪfter opening XLSTAT, select the XLSTAT / Modeling data / ANOVA command/
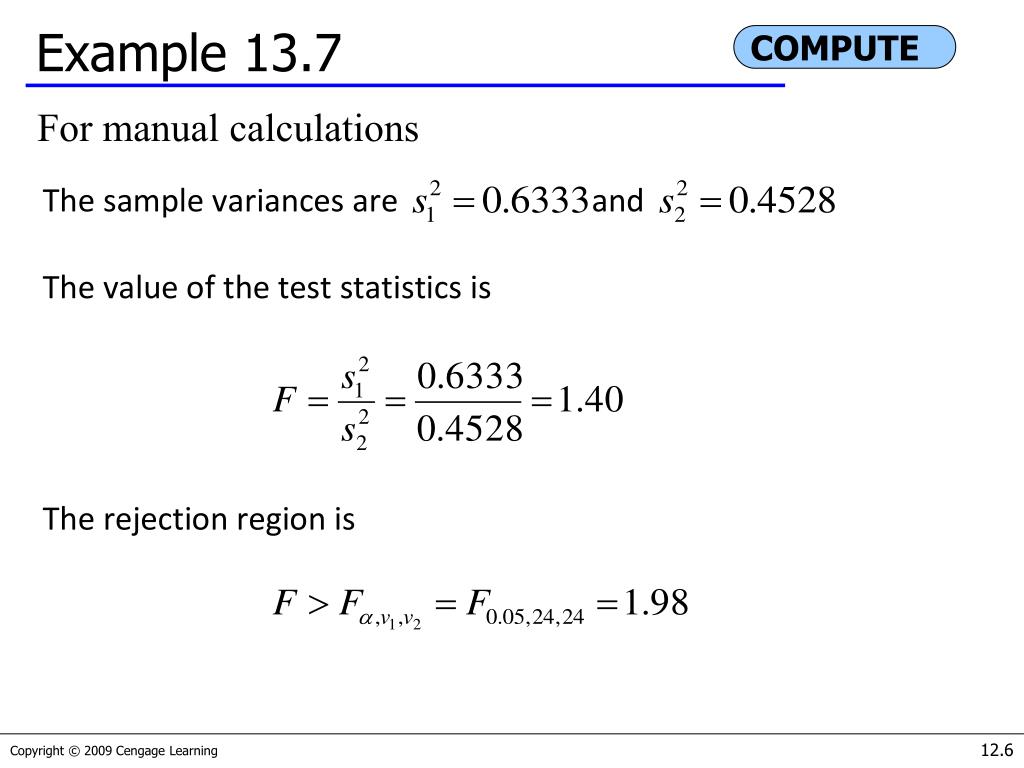
With XLSTAT's ANOVA function, we can find out if the growing method has a significant effect on the yield by controlling the type of field and the interaction between the method and the type of field. We have performed an ANOVA with interactions in order to determine the interactions between the types of methods used and the types of fields. Because the 3rd method was not tested on the 4th type of field (because of a lack of seeds), and the 2nd method on the 4th type of field (because of a hail storm), the experiment is a typical example of an unbalanced ANOVA. The yield was measured after the harvest. The data correspond to an experiment in which four different methods for growing crops were tested on four different types of fields (same soil but different light exposure). Dataset for a two-way unbalanced ANOVA with interactions Not sure this is the modeling feature you are looking for? Check out this guide. This tutorial will help you set up and interpret a two-way Analysis of Variance (ANOVA) on unbalanced data in Excel using the XLSTAT software.
